While the thought of driverless vehicles may make those of us who enjoy driving a little hesitant, it is undeniable that if they live up to expectations, one day autonomous vehicles will offer numerous potential advantages, not the least of which is the ability to reduce car crashes. Government data identifies driver behavior or error as a factor in 94 percent of crashes according to the National Highway Traffic Safety Association.
To achieve Level 5 autonomous driving (the highest level of autonomous driving), cars will need to be tested exhaustively before driving on city streets and country roads. In order to accomplish this safely, Akio Toyoda, the president of major automobile manufacturer Toyota, estimated that approximately 8.8 billion miles of testing, including simulation, are required to ensure that autonomous vehicles are safe.
It begs the question: How can automotive manufacturers expect to deploy autonomous vehicles in a reasonable amount of time to meet consumer expectations but remain safe for use?
The challenge becomes even more daunting when we look at the wide breadth of challenges that OEMs and their suppliers must address to make safe autonomous driving a reality.
Shift to electrification
Somewhat preceding and overlapping the push to autonomous driving, the ongoing shift from combustion engine-based automobiles to electrification is disrupting the automotive industry, rearranging the supply/value chain and changing how cars are architected and built. The concept of a “software-defined vehicle” opens opportunities for existing and new players to redefine and package transportation and mobility as services. At the same time, it also presents to the automotive suppliers, manufacturers and downstream customers enormous challenges with the design and verification of connected autonomous vehicles.
Complexity
The software system for a high-end vehicle is estimated to contain around 100 million lines of code, and this number is increasing. Ten times more is expected for fully autonomous operations (Levels 4/5). In contrast, the control system that runs a single U.S. military drone uses 3.5 million lines of code. Despite increasing complexity, the race toward autonomy and electrification, fueled by interests from data and consumer-centric companies such as Google, Apple, and Amazon, has intensified time-to-market pressure for automakers and OEMs.
Connectivity
Intelligent cars rely as much on external information as they do onboard sensors and cameras for safe operation. The evidence of the safety benefits from driving automation is indisputable. Driver-assisted features such as left-turn assist, look around viewing and crossing alert are integral components of the actionable artificial intelligence (AI) response loop in AVs and are paramount to safety.
Diversity
As Akio Toyoda stated, driverless cars must undergo billions of hours of training because of the unplanned conditions related to a vehicle’s surroundings.
Software and electronics, the heart of all AV self-driving systems, present both challenges and opportunities for innovation. The AV self-driving system must tailor to the specific environment into which the vehicles deploy and be continuously updated to reflect changes in the vehicle design, mechanical and electronic components, and other external factors such as the condition and availability of roadway and communication infrastructure tomeet an acceptable level of usability and accuracy. Consequently, participants in the automotive ecosystem must quickly reposition their business to adapt.
Development and verification platform
The design and verification of the connected autonomous vehicle must evolve with the shifting automotive landscape. Automotive architects must be able to effectively explore different compute and sensor options in a closed-loop environment to simulate, test and validate their design against different vehicular compute and sensor configurations, deployment options and environmental variations. Such a methodology and platform must provide verification coverage of the complete vehicular system, enable characterization of a multi-vendor ecosystem, and benchmark different key performance indicators(KPIs).
Mixed reality
A confluence of real and virtual objects that co-exist and interact in near real-time includes physical chip-level (or on-chip level) devices, SoCs, performance accelerators and even complete vehicles together with their respective virtual models and simulations. The fusion of connected automotive elements with communication infrastructure within the platform cloud elevates the depth of details and diversity of use-cases that closely mirror real-world conditions.
ISA and SoCs
Automotive components such as ADAS SoC, CPU,GPU, DSP, FPGA, VPU, Image Processor, and NPU, along with their operating software, are profoundly dependent on the instruction set architecture (ISA) chosen for them by the supplier of these components. The sensitivity of ISA to the automotive compute element cannot be overstated: cost, power, performance, reliability and security, time-to-market and competitive advantage are just a few.
Co-design
Co-design is the concept that a single language can be used to describe the platform’s hardware and software, enabling functionalities to be assigned to each as necessary to function together as a single cohesive system. In practice, the ability to evaluate the design performance under realistic workloads is fundamental to co-design and has been difficult to achieve under simulation. Software is critical to the automotive platform. The design and verification environment enables the development of software to be left-shifted in the product lifecycle to save time and facilitate design trade-offs.
Characterization
Given the complex mix of mechanical, electrical, electronic and software elements in the car, the design and verification platform provides the means for car manufacturers to evaluate the efficacy of these components from a diverse ecosystem of suppliers.
Chip to cloud
The rise of data is rapidly transforming the connected, autonomous, shared, and electric (CASE) vehicle landscape. Challenges and opportunities for automotive OEMs now extend beyond onboard components to related infrastructure and services. For example, take the concept of virtual traffic lights (VTL), which work by utilizing the car’s speed, acceleration, and distance to calculate time to green.
Conclusion
The design and verification of the future connected autonomous vehicle is a complex endeavor. Siemens and Edgelab.ai are collaborating with automotive OEMS in an open ecosystem to develop a holistic verification environment OEMs and their suppliers can use to test the reliability of their autonomous vehicle designs in a virtual environment before committing to manufacturing a vehicle.
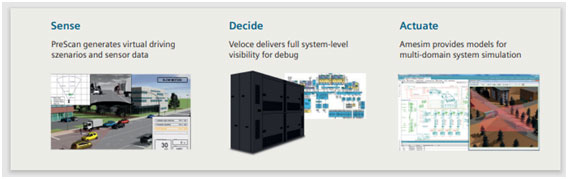